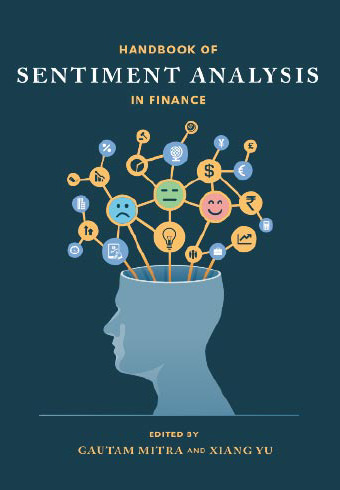
Handbook of Sentiment Analysis in Finance (2016)
Editors: Gautam Mitra and Xiang Yu
Release Date: May 2016
Available in Hardback and as an E-Book
Price: £65.00 +(P&P)
- News Wires
- Macro-economic Announcements
- Social Media
- Microblogs/Twitter
- Online (search) Information e.g. Google Trends
The applications of sentiment analysis are considered for multiple asset classes including:
- Equities
- Fixed Income Instruments
- Foreign Exchange
- Commodities (Oil, Gas, Energy and others)
- Green Commodities
Chapter 1
Progress in sentiment analysis applied to finance: an overview
Gautam Mitra (Visiting Professor, UCL; Emeritus Professor, Brunel University; CEO, OptiRisk Systems)
and Xiang Yu (Researcher, OptiRisk Systems)
Abstract: In this overview chapter we first give a summary of the different ways textual information is processed and transformed into quantitative sentiment scores. We define the concept of market sentiment and the polarity of the sentiment scores, namely, positive, negative and neutral. We delve into the roots of sentiment analysis in branding and its applications in the consumer sector. Against the backdrop of efficient market hypothesis (EMH) and the contrarian behavioural finance theories we discuss the advantages of applying sentiment data to financial markets. We consider how news stories actually affect, that is, impact the dynamical behaviour of assets as measured by price, volatility and liquidity. A number of salient meta data sources, namely, Newswires, Social Media and On-Line search results are discussed. In particular, we introduce the major attributes of meta data such that these can be used in automated applications. We also consider the financial applications which can be enhanced by Sentiment Analysis. The challenges of handling financial sentiment meta data is discussed from a statistical and institutional perspective. Finally, the sentiment meta data supplied by the major contents vendors, namely, Bloomberg, Thomson Reuters and RavenPack are explained and presented in a summary form.
Part I – TEXT ANALYTICS AND SENTIMENT CLASSIFICATION
Ch.2: Compositional Sentiment Analysis
Stephen Pulman (Professor of Computer Science, Somerville College, Oxford University; Co-founder, Thársis)
Abstract: A fundamental principle of natural language semantics is “compositionality”, the principle that the meaning of a phrase or sentence is a function of the meanings of the words contained in it and their manner of combination. This is part of the explanation of how from a finite set of word meanings it is possible to construct a potentially infinite number of distinct sentence meanings. In (Moilanen and Pulman, 2007), we argued that the sentiment polarity of a sentence is also largely compositionally derived. In the current chapter we summarise some recent developments in compositional approaches to sentiment analysis, and describe some experiments which suggest that such approaches lead to higher accuracy compared to non-compositional approaches in predicting the direction of the US non-farm payroll. These experiments are described in more detail in chapter 24 by Levenberg et al.
Ch. 3: Document Sentiment Classification
Bing Liu (Professor, Department of Computer Science, University of Illinois at Chicago)
Ch. 4: Sentence Subjectivity and Sentiment Classification
Bing Liu (Professor, Department of Computer Science, University of Illinois at Chicago)
Part II – ONLINE SEARCH AND SOCIAL MEDIA SOURCES
Ch. 5: Twitter Sentiment Analysis: Lexicon Method, Machine Learning Method and Their Combination
Olga Kolchyna (PhD Researcher, UCL), Tha´rsis Souza (PhD Researcher, UCL), Tomaso Aste (Head of Financial Computing & Analytics Group, Department of Computer Science, UCL) Philip Treleaven (Professor of Computer Science, UCL)
Abstract: This chapter covers the two approaches for sentiment analysis: i) lexicon based method; ii) machine learning method. We describe several techniques to implement these approaches and discuss how they can be adopted for sentiment classification of Twitter messages. We present a comparative study of different lexicon combinations and show that enhancing sentiment lexicons with emoticons, abbreviations and social-media slang expressions increases the accuracy of lexicon-based classification for Twitter. We discuss the importance of feature generation and feature selection processes for machine learning sentiment classification. To quantify the performance of the main sentiment analysis methods over Twitter we run these algorithms on a benchmark Twitter dataset from the SemEval-2013 competition, task 2-B. The results show that a machine learning method based on SVM and Naive Bayes classifiers outperforms the lexicon method. We present a new ensemble method that uses a lexicon-based sentiment score as input feature for the machine learning approach. The combined method proved to produce more precise classifications. We also show that employing a cost-sensitive classifier for highly unbalanced datasets yields an improvement in sentiment classification performance of up to 7%.
Ch. 6: Sentiment Analysis in Microblogs
Federico Pozzi (Currently: Analytical Consultant, SAS; Formerly: Researcher, University of Milano-Bicocca), Enza Messina (Professor, University of Milano-Bicocca), Elisabetta Fersini (Postdoctoral Research Fellow, University of Milano-Bicocca)
Abstract: The huge amount of textual data on the Web has grown in the last few years rapidly creating unique contents of massive dimensions that constitutes fertile ground for Sentiment Analysis (SA). In particular, microblogs represent an emerging challenging sector where the natural language expressions of people can be easily reported through short but meaningful text messages. Since behavioural economics tells us that emotions can profoundly affect individual behaviour and decision making, this unprecedented contents of huge dimensions need to be efficiently and effectively analysed. A key information that can be grasped from social environments relates to the polarity of text messages, i.e., the sentiment (positive, negative or neutral) that the messages convey, useful to predict changes in various economic and commercial indicators. The growing interest of SA is related to the possibility of exploiting its results in different tasks, such as: understand and forecast the sentiment of financial markets (Mitra and Mitra, 2011), manage business intelligence tasks related to users feedback (Pang and Lee, 2008) or sound out public opinion during political campaigns (O’Connor et al., 2010).
In this chapter, the literature review regarding SA applied to microblogs using supervised and semi-supervised models is presented. Most of the works consider text as unique information to infer sentiment, do not taking into account that microblogs are actually networked environments. A representation of real world data where instances are considered as homogeneous, independent and identically distributed (i.i.d.) leads us to a substantial loss of information and to the introduction of a statistical bias. For this reason, the combination of content and relationships is a core task of the recent literature on Sentiment Analysis.A further and interesting aspect we present concerns models and techniques for sarcasm and irony detection in microposts. In this setting we present different works which leverage additional features in the bag-of-words model which are symptomatic of a greater emphasis in sentiment (Part-Of-Speech, Emoticons, Expressive lengthening, etc.).
Ch. 7: Quantifying Wikipedia Usage Patterns before Stock Market Moves
Helen Susannah Moat (Associate Professor of Behavioural Science, Warwick Business School, Warwick University), Chester Curme (Currently: Quantitative Analyst, Loomis, Sayles and Company; Formerly: Research Assistant, Boston University), Tobias Preis (Associate Professor of Behavioural Science & Finance, Warwick Business School, Warwick University; Founder, Artemis Capital Asset Management), H. Eugene Stanley (Distinguished Professor, Boston University), Adam Avakian (Boston University), Dror Y. Kenett (Currently: Researcher, US Department of Treasury, OFR; Formerly: Researcher, Boston University).
Abstract: Financial crises result from a catastrophic combination of actions. Vast stock market datasets offer us a window into some of the actions that have led to these crises. Here, we investigate whether data generated through Internet usage contain traces of attempts to gather information before trading decisions were taken. We present evidence in line with the intriguing suggestion that data on changes in how often financially related Wikipedia pages were viewed may have contained early signs of stock market moves. Our results suggest that online data may allow us to gain new insight into early information gathering stages of decision making.
Ch. 8: Investor Attention and the Pricing of Earnings News
Asher Curtis (Assistant Professor and Herbert O. Whitten Endowed Professorship in Accounting, University of Washington), Vernon J. Richardson (Professor in Accounting, University of Arkansas) & Roy Schmardebeck (Assistant Professor, University of Missouri)
Abstract: We investigate whether investor attention is associated with the pricing (and mispricing) of earnings news where investor attention is measured using social media activity. We find that high levels of investor attention are associated with greater sensitivity of earnings announcement returns to earnings surprises, with the effect being strongest for firms that beat analysts’ forecasts. This appears to be appropriate pricing, on average, as only firms with low levels of attention are associated with significant post-earnings-announcement drift. Our results are distinct from other information sources including traditional media outlets, financial blogs, and internet search engine activity. Our results are consistent with investor attention observed in social media activity having distinct effects on the pricing and mispricing of earnings news.
PART III – SENTIMENT ANALYSIS APPLIED TO EQUITIES
Ch. 9: Predicting Stock Returns using Text Mining Tools
Gurvinder Brar (Global Head of Quantitative Research, Macquarie Securities), Giuliano De Rossi (Head of European Quantitative Research Team, Macquarie Securities) & Nilesh Kalamkar (Quantitative Researcher, Macquarie Securities)
Abstract: This paper documents the research undertaken by the Macquarie Global Quantitative Research Group utilising text mining tools applied to ‘unstructured text’ to predict stock returns. We focus on announcements relating to publication of company reports and earnings press releases. Moreover, we discuss directions for future research that are likely to be of particular relevance for practitioners. Our main conclusion is that investors should utilise text mining tools within their investment process. These tools can help extract information embedded within corporate filing data and the slower decay of the predictive ability of the resulting signal makes them particularly suitable for investors with longer investment horizons.
Ch. 10: Sentiment and Investor Behaviour
Elijah DePalma (Senior Quantitative Research Analyst, Thomson Reuters)
Abstract: Thomson Reuters News Analytics provides automated sentiment and linguistic analytics on financial news, and using Thomson Reuters News Analytics we construct a US market sentiment index from corporate Reuters news sentiment. Following recent literature identifying the pervasive influence of market sentiment on market anomalies and on the pricing of risk factors, we use this US market sentiment index to demonstrate the influence of market sentiment on the post-earnings announcement drift anomaly, the accruals anomaly, and market risk premium. We show that the classic risk-return trade-off of the Capital Asset Pricing Model (CAPM) holds following negative market sentiment periods, whereas the underperformance of high-risk securities known as the low-volatility anomaly holds following positive market sentiment periods. Thus, we propose a dynamic methodology which accepts the risk-return relationship of either the CAPM or the low-volatility anomaly following periods of negative or positive market sentiment, respectively. We further demonstrate the influence of market sentiment on the earnings revisions anomaly. As an application we present a monthly quant factor timing strategy driven by market sentiment, and improve upon this strategy by implementing the above, proposed dynamic methodology.
Ch. 11: Thematic Alpha Streams Improve Performance of Equity Portfolios
Peter Hafez (Chief Data Scientist,, RavenPack)
Abstract: In this paper, we propose a robust methodology for equity portfolio construction using news-based thematic alphas. These alphas are the result of our previous research, where we took advantage of RavenPack´s event taxonomy to build a set of theme-based sentiment indicators. Now, we develop the idea further and combine a large set of thematic alpha streams into an overall equity portfolio. In general, we find that employing our methodology in a long/short strategy yields strong return and turnover improvements, versus treating sentiment as one-dimensional, over our 8-year backtesting period, across both region (Europe and US) and size (small, mid, and large market capitalization stocks).
Ch. 12: The Psychology of Markets: Information processing and the impact on asset prices
Richard Peterson (CEO, MarketPsych)
Abstract: Crowds move markets. Such crowds are made up of individuals – individuals who invest, trade, or manage portfolios. They are moved not only by what they read and hear, but often more so by their emotional reactions to such information. Behavioural economics researchers have demonstrated that when new information provokes emotional responses such as joy, fear, anger, and gloom, individual trading behaviours are systematically biased. And since individuals combine to form a market, their collective emotions manifest in observable market behaviour. This paper reviews the literature on how specific psychological stimuli impact information processing and trading behaviour. The paper uses a cross-sectional rotation model to demonstrate empirical evidence of the possibility of information arbitrage in equity markets, with an emphasis on the value of anger and leadership trust.
Ch. 13: An Impact Measure for News: its use in (daily) trading strategies
Gautam Mitra (Visiting Professor, UCL; Emeritus Professor, Brunel University; CEO, OptiRisk Systems), Xiang Yu (Researcher, OptiRisk Systems), Cristiano Arbex-Valle (Senior Software Engineer and Consultant, OptiRisk Systems) and Tilman Sayer (Senior Quant Research Analyst, OptiRisk Systems)
Abstract: We investigate how ‘news sentiment’ in general and the ‘impact of news’ in particular can be utilised in designing equity trading strategies. News is an event that moves the market in a small way or big. We have introduced a derived measure of news impact score which takes into consideration news flow and decay of sentiment. Since asset behaviour is characterised by return, volatility and liquidity we first consider a predictive analytic model in which market data and impact scores are the inputs and also the independent variables of the model. We finally describe the trading strategies which take into consideration the three important characteristics of an asset, namely, return, volatility and liquidity. The minute-bar market data as well as intraday news sentiment metadata have been provided by Thomson Reuters.
Ch. 14: The Unbearable Lightness of Expectations of the Chinese Investor
Eric Tham (Director of Quantitative Strategies, iMaibo)
Abstract: The Chinese equities market have witnessed wild swings in 2014-2015. Its impact on the Chinese economy and in turn on the Feds perpetual decision to raise rates has been indirect but substantial The high internet penetration of the Chinese population – about 670 million and the large increase of its new retail trading accounts makes it conducive for investor herding. In this paper, investor sentiment is separately derived through the textual analysis of newswires and the social blogs, which reacts those of rational arbitrageurs and retail noise traders. Through a state space model of index returns on the two types of sentiment, it is shown that social blog sentiment and its time varying sensitivities are most accountable for the index swings in 2014/15. Whilst this sensitivity to the blog sentiment has since decreased in June 2015 leading to a more stable stock market, it remains to be seen if the market is less sentiment driven now.
PART IV – SENTIMENT ANALYSIS FOR OTHER ASSET CLASSES: ENERGY, COMMODITIES, GREEN COMMODITIES, BONDS, AND FX
Ch. 15: The Role of News in Commodity Markets
Svetlana Borovkova (Associate Professor, Vrije Universiteit Amsterdam; Researcher, Dutch Central Bank)
Abstract: In this chapter, we give a broad overview of how commodity-related news affect commodity markets. We examine the main commodity classes: energy, agriculturals and metals, as well as various ways markets respond to news: in terms of prices, returns, volatilities and price jumps. Market responses are analysed for different latencies, ranging from minutes to days and to longer horizons. We discuss how these insights can be used in trading strategies, investment decisions and risk management.
Ch. 16: Predicting Global Economic Activity with Media Analytics
Richard Peterson (CEO, MarketPsych), Aleksander Fafula (Chief Data Scientist, MarketPsych)
Abstract: In this paper we demonstrate how real-time news and social media analytics can be used to model global economic activity. Accurately quantifying economic growth in a timely fashion is an enduring challenge to economists. With the advent of big data and real-time information, data that reflects economic activity is now available via a variety of nearly instantaneous sources. Internet search character and volume (Google Trends), credit card transaction histories (e.g., Visa transaction data), and quantified news and social media content (e.g., Thomson Reuters MarketPsych Indices) are all varieties of such information. The Thomson Reuters MarketPsych Indices (TRMI) – the subject of this paper – are quantified sentiment and macroeconomic time series derived from the flow of news and social media information about individual locations and countries. In this paper we demonstrate how data derived from news and social media analytics can be used to model and predict daily economic activity in individual countries. Our research finds that predictive models built with the TRMI data show outstanding in-sample and forward-tested accuracy in predicting real-time economic activity (the PMI) for the G-12 nations.
Ch. 17: Credit Risk Assessment of Corporate Debt using Sentiment and News
Dan diBartolomeo (Founder, Northfield Information Services)
Abstract: Since the Global Financial Crisis of 2007–2009, history has been marked by numerous failures to correctly assess the credit worthiness of financial instruments, financial institutions and governments. Institutional confidence in the traditional credit rating agencies has been greatly reduced. One of the largest rating agencies, Standard and Poors, recently agreed to pay a $1.4 billion fine to US regulators for alleged widespread negligence in the rating of certain complex financial instruments. As an alternative to the traditional rating process, this work will illustrate the potential use of sentiment statistics from quantified news to calibrate and update the credit risk of corporations and financial institutions in real time. A modified version of the Merton (1974) contingent claims model from diBartolomeo (2010, 2012) is used to break each corporate debt into two pieces, the first considered riskless debt and the second equity in the issuer. We utilize news flows and sentiment statistics to frequently update the expected volatility of the assets of the firm and hence the credit risk of the debt in terms of both the probability of default and loss given default.
Ch. 18: Trading Bond Futures (& FX) with News Meta Data
Saeed Amen (Managing Director and Co-founder, The Thalesians)
Abstract: Over the past few years, strategies which use news analytics have become more popular. Whilst the focus has been on equities, there is also significant news flow when it comes to macro assets. Here, we examine how macro news analytics data can be used to trade bond futures (& FX). We create news-based economic sentiment indices (NBESI) which mimic the behaviour of growth surprise indices. We discuss more broadly the relationship between growth surprise indices, NBESI and bond markets.We use these news indices to create trading rules for bond futures. Our NBESI bond futures basket has risk-adjusted returns of 1.14 and drawdowns of 7.7% since 2001, outperforming a passive basket with risk-adjusted returns of 0.79. Our NBESI UST futures spreads basket has risk-adjusted returns of 0.90 which outperforms a passive strategy with risk-adjusted returns of 0.46. We also apply the same approach to trading FX, using news data. Our combined filtered G10 FX carry and G10 FX NBESI basket has risk-adjusted returns of 1.11 and drawdowns of 6.7%.
Ch. 19: Currency Sentiment Analysis
Richard Peterson (CEO, MarketPsych) , Changjie Liu (Chief of Analytics, MarketPsych)
Abstract: Alexander Hamilton identified that uncertainty irrationally debases a currency and trust inflates its value. Researchers have since found that monetary policy uncertainty adds a risk premium (an excessive discount) in currency values. Media sentiment gauges perceptions, and as such it holds promise toward the identification of currencies with relatively larger risk premia. Using a unique array of media-derived currency sentiment data – the Thomson Reuters MarketPsych Indices (TRMI), the authors demonstrate that colloquial wisdom about the drivers of currency valuations may be supported by such data. In particular, the Uncertainty TRMI shows significant historical predictive value over currency valuations in cross-sectional models at weekly and yearly horizons, likely due to investor overreaction to uncertainty. Media price forecasts and expressed trust also appear to hold predictive value. Moving average crossovers (MACDs) may help time reversals in influential information flow, as in the case of the Japanese yen priceForecast TRMI. Using a combination of orthogonal TRMI boosts model returns in sample.
PART V – USE OF SENTIMENT ANALYSIS IN WEEKLY, DAILY AND HIGH FREQUENCY TRADING
Ch. 20: Role of Options Markets in Price Discovery: Trading around News on Dow 30 Options
Nitish Sinha (Economist, Federal Reserve Board), Wei Dong (Credit Risk Analyst Lead, AIG)
Abstract: Using intraday data on stocks, options and firm-specific news events for Dow30 stocks, we find the volume of trading in the options increases almost seven times an hour before news, whereas the stock volume increases by 17%. Since the trading in the option market spikes prior to news, it is probably a venue for informed trading. Trading in the option market continues to be at elevated level well after the news, suggesting traders with disagreement also prefer to trade in the option market. The results suggest that options are important for price discovery due to informed as well disagreement-induced trading.
Ch. 21: Abnormal news volume and underreaction to soft information.
Michal Dzielinski (Postdoctoral Research Fellow, Stockholm University)
Abstract: News tone has been gaining popularity in the academic literature as a measure “soft information” and numerous studies have explored its role for asset prices. . However, as far as tone can give a good indication of whether the content was positive or negative, it does not tell anything about the importance of the announcement. I propose a measure of importance, abnormal news volume, and interact it with tone to examine subsequent stock returns. I find significantly more drift after highly publicized announcements, suggesting important news takes longer to incorporate into prices. The results are stronger for negative than for positive stories.
Ch. 22: Automated Analysis of News to Compute Market Sentiment: Its Impact on Liquidity and Trading
Gautam Mitra (Visiting Professor, UCL; Emeritus Professor, Brunel University; CEO, OptiRisk Systems), Xiang Yu (Researcher, OptiRisk Systems), Dan diBartolomeo (CEO, Northfield; Visiting Professor, Brunel University) and Ashok Banerjee (Financial Research and Trading Lab, IIM Calcutta)
Abstract: Computer trading in financial markets is a rapidly developing field with a growing number of applications. Automated analysis of news and computation of market sentiment is a related applied research topic which impinges on the methods and models deployed in the former. In this review we have first explored the asset classes which are best suited for computer trading. We present in a summary form the essential aspects of market microstructure and the process of price formation as this takes place in trading. We critically analyse the role of different classes of traders and categorise alternative types of automated trading. We introduce alternative measures of liquidity which have been developed in the context of bid-ask of price quotation and explore its connection to market microstructure and trading. We review the technology and the prevalent methods for news sentiment analysis whereby qualitative textual news data is turned into market sentiment. The impact of news on liquidity and automated trading is critically examined. Finally we explore the interaction between manual and automated trading.
PART VI – APPLICATIONS OF SENTIMENT ANALYSIS: CASE STUDIES
Ch. 23: Twitter Sentiment Analysis Applied to Finance: A Case Study in the Retail Industry
Tha´rsis Souza (PhD Researcher, UCL), Olga Kolchyna (PhD Researcher, UCL) and Tomaso Aste (Head of Financial Computing & Analytics Group, Department of Computer Science, UCL)
Abstract: This chapter presents a financial analysis over Twitter sentiment analytics extracted from listed retail brands. We investigate whether there is statistically-significant information between the Twitter sentiment and volume, and stock returns and volatility. Traditional newswires are also considered as a proxy for the market sentiment for comparative purposes.The results suggest that social media is indeed a valuable source in the analysis of the financial dynamics in the retail sector sometimes carrying larger prior information than mainstream news such as The Wall Street Journal and Dow Jones Newswires.
Ch. 24: Financial Prediction from Heterogenous Streams of Online Lead Indicators
Abby Levenberg (Formerly: Senior Research Assistant, Oxford-Man Institute, University of Oxford; Currently: Research Scientist, WorkFusion), Stephen Pulman (Professor of Computer Science, Somerville College, Oxford University; Co-founder, Thársis), Edwin Simpson (Postdoctoral Research Fellow, Oxford University), Stephen Roberts (Professor of Machine Engineering, Oxford University), Karo Moilanen (Co-founder & CTO, Thársis) and Georg Gottlob (Professor of Computing Science, Oxford University)
Abstract: Learning to predict trends of financial and economic variables is a hard problem with a large body of literature devoted to it. Further, companies and sources that provide financial and economic data do so at a premium. As such, there is a significant amount of work on using freely available sources of big text data from the WWW to learn from. Much of this work has relied on some form or other of superficial sentiment analysis to generate the text features for the learners. In this project report we extend the current literature and present a framework for learning from Streams of Online Lead Indicators (SOLID). We describe a novel approach for economic prediction using heterogeneous streams of Web data. We incorporate different data types into our model – such as time series and text – by treating each data stream as an independent source with its own features and posterior distribution. For the text data streams we use a novel approach to prediction using a sentiment composition model to generate features that can operate over much lower levels of granularity than in the prior literature. We then use a Bayesian classifier combination model to combine the suite of independent “weak” predictions into a single prediction of the primary economic and financial variables. We report experiments over multiple instruments and time frames including daily versus monthly trends. Our results show that the SOLID can achieve high predictive accuracy for a variety of leading indicators.
PART VII – Directory of Service Providers
- Alexandria
- Amareos
- Bloomberg
- Deltix
- iMaibo
- Lamplight Analytics
- MarketPsych
- Northfields
- OptiRisk Systems
- Psychsignal
- RavenPack
- Stocktwits
- Thomson Reuters
Use the Online Order Form
PHONE your credit card order:
+44 (0) 1895 256 484
You can also use the order form in the flyer and submit it one of the following ways:
EMAIL:
info@optirisk-systems.com
Sponsored by
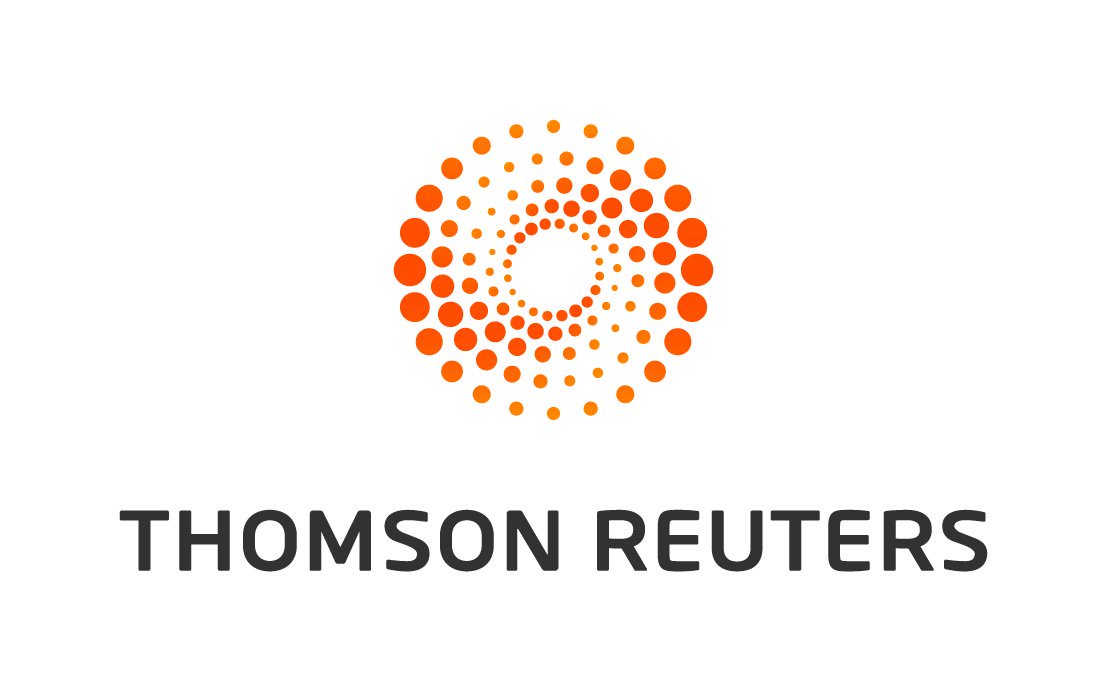
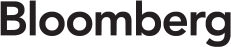


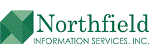