Handbook of Alternative Data in Finance, Volume I (CRC Press/OptiRisk Series in Finance)
Released: 12 July 2023 Hardcover
Price: £50.00 + (P&P £5)
Cutting-edge applications in machine learning, fintech, and more, the handbook caters to quantitative analysts, postgraduates, financial mathematics researchers, and other market participants. Featuring contributions from prominent experts, it offers a 360-degree view of Alternative Data’s role in predicting market trends, guiding investments, and managing risks.
OptiRisk’s USP is research, knowledge acquisition and sharing, in the domain of News Analytics, Sentiment Analysis and Alternative Data in Finance. Our journey started with The Handbook of News Analytics in Finance (2011), which was followed by The Handbook of Sentiment Analysis in Finance (2016). We have stayed the course, and as a Financial Analytics company continued to research in the domain of trading and fund management; this has culminated in our latest work: The Handbook of Alternative Data in Finance (2023), a vital resource in an era where data-driven decisions reign supreme. Unlike single-authored works, this collaborative effort provides a diverse and comprehensive perspective on Alternative Data’s implications.
In the ever-evolving landscape of data’s ascendancy, this handbook serves as a compass, guiding financial professionals and academics through the intricate relationship between data and decision-making. This handbook is an indispensable guide to informed decision making and creating trading and fund management strategies.
Product details
Publisher: CRC Press/OptiRisk Series in Finance; 1st edition (12 July 2023)
Language: English
Hardcover: 576 pages
Chapter 1. Alternative Data: Overview
Gautam Mitra (Research Director, OptiRisk Systems and UCL Department of Computer Science, London, United Kingdom), Kieu Thi Hoang (Senior Financial Analyst, OptiRisk Systems), Alexander Gladilin (Research Associate, OptiRisk Systems), Yuanqi Chu (Sponsored PhD Candidate and Intern, OptiRisk Systems), Keith Black (Managing Director and Program Director, FDP Institute), Ganesh Mani (Adjunct Faculty, Carnegie Mellon University)
ABSTRACT
In this overview chapter we first discuss the importance and growth of data, in many application domains. Data as used in modelling, that is, analytics are considered from multiple perspectives; these (multiple) data views apply equally to traditional data and alternative data. Alternative data in some sense, has emerged out of big data and in many contexts, these can manifest as large unstructured datasets. In this Handbook we focus mainly on financial applications, indeed many financial (expert) practitioners have been using alternative data to create trading strategies, investment strategies and risk management applications. Acquiring or having access to alternative data has emerged as an important issue. A new genre of market participants has appeared in the financial scene, namely alternative data brokers/vendors. These alternative data vendors aggregate data from diverse sources; for analysts who wish to use these alternative data they provide (i) legal framework for access and (ii) if required some exemplar models to use such datasets.
PART (I) ALTERNATIVE DATA: PROCESSING AND IMPACT
Chapter 2. Contemplation and Reflection on Using Alternative Data for Trading and Fund Management
David Jessop (Columbia Threadneedle Investments)
ABSTRACT:
Alternative data (together with machine learning) is the topic du jour for many quants and data scientists. These quantitative professionals want to get their hands on some new datasets and try a new machine learning technique on the data. In this chapter we reflect on this process. We do not focus on the exact approach to take, nor do we analyse a particular dataset. Instead, we hope to give some guidance around not only building models with alternative data but the work that has to go in to changing these models from a prototype into a production ready system which can be run day-to-day.
Chapter 3. Global Economy and Markets Sentiment Model
Jacob Gelfand (Northwestern Mutual, Investment Risk Management), Kamilla Kasymova (Northwestern Mutual, Investment Risk Management), Seamus O’Shea (Northwestern Mutual, Managed Investments), Weijie Tan (Northwestern Mutual, Investment Risk Management)
ABSTRACT
Investment research involves collecting diverse information from various sources, and piecing these together to form a mosaic; this mosaic is then used to obtain a view of the risks and opportunities in the market or within an asset class. The task is laborious, prone to bias and human error, and naturally limited in its scope. In our implementation of the Global Economy and Markets Sentiment (GEMS) model we have considered news sentiment analysis, and have addressed these shortcomings. We have reached into previously untapped information sources, and employed the tools of AI and Machine Learning, and power computing to manage scale while mitigating errors and biases. In this paper we describe the model’s core framework and implementation, and the dataset upon which it was built. We propose different use cases and explore several investment strategies; this work is based on insights obtained by us through our continuing and sustained research . The model was conceived and developed within the Managed Investments department at the Northwestern Mutual Life Insurance Company, specifically by the department’s Quantitative Research and Strategy team. In its current application, the GEMS model helps the Emerging Markets investment team gain insights from large volumes of global and local news in over 65 languages. The principal objective is to reach better and informed investment decisions, and to achieve these before the market moves.
PART (II) COUPLING MODELS WITH ALTERNATIVE DATA FOR FINANCIAL ANALYTICS
Chapter 4. Enhanced Corporate Bond Yield Modelling Incorporating Macroeconomic News Sentiment
Zhixin Cai (OptiRisk Systems Ltd, London, United Kingdom), Christina Erlwein-Sayer (Financial Mathematics, University of Applied Sciences HTW Berlin, Berlin, Germany), Gautam Mitra (CEO, OptiRisk Systems Ltd and UCL Department of Computer Science, London, United Kingdom)
ABSTRACT
In this study, we assess dynamics of credit spreads from corporate bonds by involving news sentiment data together with historical market data . Typically, a higher yield spread is associated with higher credit risk. By predicting the upward/downward movement of yield and yield spread accurately, the creditrisk associated to the bonds can be detected precisely. The corporate bonds studied are issued after 1 January 2007 by seven chosen companies listed in Euro Stoxx 50 index. The time series of bond yields and news sentiment cover the period from 1 January 2007 to 15 May 2017. The modelling of the dynamics of corporate bond yields and credit spreads are based on ARIMA and ARIMAX models. In the ARIMAX model, macroeconomic and firm-specific news sentiment are used as the external explanatory variable. We examine the effect of several categories of macroeconomics news sentiment and firm-specific news sentiment on corporate bond yield spreads. Furthermore, we separate positive and negative sentiment and investigate their impact on the forecast of corporate bond yields. It is found that negative country news sentiment and central bank news sentiment are effective during a recession period and positive country news sentiment is effective in the recovery period. Negative government and firm-specific news sentiment, in general, affect corporate bond yield spreads more than positive government and company news sentiment.
Chapter 5. AI, Machine Learning and Quantitative Models
Gautam Mitra (OptiRisk Systems and UCL Department of Computer Science, London, United Kingdom), Yuanqi Chu (OptiRisk Systems), Arkaja Chakraverty (OptiRisk Systems), Zryan Sadik (OptiRisk Systems)
ABSTRACT
This chapter has been set out in the style of a tutorial and provides an overview of some common features of Neo-Classical Quant (NCQ) models and newly emerging paradigms of Artificial Intelligence and Machine Learning (AI & ML) models. Models are used for contemplation, abstraction, and creative thinking. Models can broadly be classified into three types: Linguistic, Mathematical, and Computer models. The central concept of Modelling is best explained using a taxonomy or categorization of models. This taxonomy is based on four paradigms of Descriptive, Normative, Prescriptive, and Decision Models. In the domain of finance, the role of time and uncertainty analysis is seen to be paramount. Any ex-ante decision such as trading, or portfolio choice has to take into account future outcomes. Hence, management scientists have been preoccupied with investigating two central challenges, namely, decisionmaking and predicting. We have chosen a simple problem that requires a directional prediction. We use NCQ and AI & ML models to predict the directional movement of VIX, that is, whether VIX closes above or below the level of the previous day’s close.
PART (III) HANDLING DIFFERENT ALTERNATIVE DATASETS
Chapter 6. Asset Allocation Strategies: Enhanced by Micro-Blog
Zryan Sadik (OptiRisk Systems Ltd, London, United Kingdom), Gautam Mitra (OptiRisk Systems Ltd and UCL, Department of Computer Science, London, United Kingdom), Shradha Berry (OptiRisk Systems Ltd, London, United Kingdom), Diana Roman (OptiRisk Systems Ltd and Brunel University London, Department of Mathematics, London, United Kingdom)
ABSTRACT
The rapid rise of social media communication has touched upon all aspects of our social and commercial life. In particular, the rise of social media as the most preferred way of connecting people on-line has led to new models of information communication amongst the peers. Of these media Twitter has emerged as a particularly strong platform and in the financial domain tweets by market participants are of great interest and value. News in general, and commercial and financial news wires, in particular provide the market sentiment and in turn influence the asset price behaviour in the financial markets. In a comparable way micro-blogs of tweets generate sentiment and has an impact on market behaviour, that is, the price as well as the volatility of stock prices. In our recent research we have introduced news sentiment based filters such as News RSI (NRSI) and Derived RSI (DRSI), which restrict the choice of asset universe for trading. In this present study, we have extended the same approach to StockTwit’s data. We use the filter approach of asset selection and restrict the available asset universe. We then apply our daily trading strategy using the Second Order Stochastic Dominance (SSD) as an asset allocation model. Our trading model is instantiated by two time series data, namely, (i) historical market price data and (ii) StockTwits sentiment (scores) data. Instead of NRSI we compute the Micro-blog RSI (MRSI) and using this a DRSI is computed. The resulting combined filter (DRSI) leads to an enhancement of the SSD based trading and asset allocation strategy. Empirical experimental results of constructing portfolios are reported for S&P 500 Index constituents.
Chapter 7. Asset Allocation Strategies: Enhanced by News
Zryan Sadik (OptiRisk Systems Ltd., London, United Kingdom), Gautam Mitra (OptiRisk Systems and UCL Department of Computer Science, London, United Kingdom), Ziwen Tan (OptiRisk Systems Ltd., London, United Kingdom), Christopher Kantos (Alexandria Technology, New York, United States), Dan Joldzic (Alexandria Technology, New York, United States)
ABSTRACT
The explosive development of electronic media has brought to the market participants thousands of pieces of financial news which are released on different platforms every day. Many news wires published online are editorially controlled and can be relied as factual summary as opposed to fake news or disinformation. These news items provide a rich source of textual information which in a summative way represents the sentiment of the market. The sentiments influence or impact the asset price as well as the volatility of individual assets. In this study we have tested sentiment enhanced daily trading strategies. Alexandria Technology has provided us news sentiment metadata, which is used in this study. We have also resorted to ‘asset filters’ which we use to restrict the universe of assets chosen for daily trades. We have considered quantified news sentiment and its impact on the movement of asset prices as a second time series data, which is used together with the asset price/return time series data. Our asset allocation strategy uses Second Order Stochastic Dominance (SSD); see . Following this modelling paradigm we compute daily trade schedules using a time series of historical equity price data. In contrast to classical mean-variance method this approach improves the tail risk as well as the upside of the return. In our recent research we have introduced news sentiment indicators such as News RSI (NRSI) and Derived RSI (DRSI) filters. These filters restrict the choice of asset universe for trading. Consistent performance improvement achieved in back-testing vindicates our approach.
Chapter 8. Extracting Structured Datasets from Textual Sources – Some Examples
Matteo Campellone (Executive Chairman and Head of Research, Brain), Francesco Cricchio (CEO and CTO, Brain)
ABSTRACT
We hereby present some examples of information extraction from textual sources such as news, company regulatory filings or earning calls transcripts. For the company filings we refer to some recent literature arguing the existence of unexploited information in these documents. We present three Brain datasets that provide several measures on various textual sources with well-defined time-stamps, and that can be input to quantitative investment models.
Chapter 9. Comparative Analysis of NLP Approaches for Earnings Calls
Christopher Kantos (Alexandria Technology, London, United Kingdom), Dan Joldzic (Alexandria Technology, London, United Kingdom), Gautam Mitra (OptiRisk Systems and UCL Department of Computer Science, London, United Kingdom), Kieu Thi Hoang (OptiRisk Systems, London, United Kingdom)
ABSTRACT
The field of natural language processing (NLP) has evolved significantly in recent years. In this chapter we consider two leading and well-established methodologies, namely, those due to Loughran McDonald, and FinBERT. We then contrast our approach to these two approaches and compare our performance against these methods which are considered to be benchmarks. We use S&P 500 market data for our investigations and describe the results obtained following our strategies. Our main consideration is the Earnings Calls for the S&P 500 stocks. We vindicate our findings and present the performance of our trading and fund management strategy which shows better results.
Chapter 10. Sensors Data
Alexander Gladilin (OptiRisk Systems), Kieu Thi Hoang (OptiRisk Systems), Gareth Williams (Transolved Ltd), Zryan Sadik (OptiRisk Systems)
ABSTRACT
Alternative data is data from non-traditional sources that can be used when making financial decisions. The success in the application of alternative data to finance means that businesses and traders are looking at such data and their applications to gain further insights. Sensors on all scales are used to obtain alternative data, from satellites in orbit which measure activity to personal devices determining location. This paper provides examples of sensor data and how it can be applied to the business world.
An introduction to sensor data is given and its different categories examined. Examples of satellite, geolocation and weather data are presented with an overview of current work and literature with an introductory methodology on how such data can be used in each case. An investigation is presented into classification techniques used for counting cars from satellite data and the application of such techniques to business decisions.
Geolocation data and its use in measuring consumer traffic both digitally and physically is examined using tools such as Google Gears and its application to financial decision making, for example by hedge fund managers, is discussed. Sensors can also be used to measure foot traffic at physical business locations. Visual sensors can be used to generate a 3D map to measure the volume of customers with 90% accuracy.
The collection of weather data supports models in areas such as forecasting crop yield and calculating weather derivatives. An overview of current techniques is presented, how statistical models are formed from such data and how these models are applied.
Additional sources of alternative data and their potential uses are presented to further demonstrate the wide applicability of sensor data. Further works and references are highlighted at the end.
PART (IV) ALTERNATIVE DATA USE CASES IN FINANCE
(PART IV.A) APPLICATION IN TRADING AND FUND MANAGEMENT (Finding New Alpha)
Chapter 11. Media Sentiment Momentum
Anthony Luciani (MarketPsych Data), Changjie Liu (MarketPsych Data), Richard Peterson (MarketPsych Data)
ABSTRACT
Media reports can have both instantaneous and delayed impacts on stock prices. Using the aggregated financial news and social media-based sentiment scores from the Refinitiv MarketPsych Analytics dataset on Russell 3000 index constituents from 2006 to 2020, a strong sentiment-related momentum, driven by investor under-reaction is identified. The characteristics of sentiment momentum in both bull and bear markets and across global regions are established; consistent with price-momentum, stocks rebounding from a bear market experience a reversal in their sentiment momentum. Time aggregations from one day to one year are characterized by this effect. Sentiment momentum is present across global regions. Controls for price momentum, value, and other fundamental models demonstrate that sentiment momentum is highly correlated with price momentum after the first month. Granger causality analysis finds that sentiment momentum is causal of price momentum.
Chapter 12. Defining Market States with Media Sentiment
Tiago Quevedo Teodoro (MarketPsych Data), Joshua Clark-Bell (MarketPsych Data), Richard L. Peterson (MarketPsych Data)
ABSTRACT
In this study, we looked for a strategy that would rotate between an equity-based portfolio and a bond-based portfolio given the properties of hidden market regimes. We used end-of-the-day price data for the SPY (S&P 500) and AGG (U.S. Bond) ETFs and MarketPsych sentiment data as features. The evaluated period was from January 1998 to September 2021. In-sample results (1998-2005) indicated that states with a negative SPY expected return are, on average, only associated with a realised negative 1-day forward return when the contemporaneous sentiment is also negative. We translated this finding in the validation period (2006-2015) with a strategy that rotates from 100% long SPY into 100% long AGG when in such state. The outperformance of the strategy (vs the SPY buy& hold) in the validation period was confirmed in the out-of-sample period (2016-2021). The back-tested portfolio yielded a theoretical Sharpe ratio of 1.3 (versus a 0.9 of the long-only SPY).
(PART IV.B) APPLICATION IN RISK CONTROL
Chapter 13. A Quantitative Metric for Corporate Sustainability
Dan diBartolomeo (Northfield Information Services), William Zieff (Northfield Information Services)
ABSTRACT
In recent years the concept of the “sustainability” companies has been at the forefront of concerns for many investors.As most consideration of sustainability has focused on the broad ideas of ESG concerns (Environmental, Social, Governance) the field has lacked a straightforward metric by which investors can assess “How many years into the future is a given company likely to survive without bankruptcy?”. While somewhat similar to a credit rating, such a measure must also consider the situation of firms with no current debt, and those that are pathologically conservative so as to survive until eventually becoming obsolete. Such a metric was introduced in based on an extension of the Merton contingent-claims model . In this study, we illustrate refinements of the methodology and present empirical analysis of the relationship between the sustainability metric and investor returns from 1992 through 2021 for all equities traded on US exchanges (inclusive of nonUS firms traded in ADR form). The results show statistically significant relationships that may be exploited for superior returns in both equity and corporate bond markets.
Chapter 14. Hot off the Press: Predicting Intraday Risk and Liquidity with News Analytics
Ryoko Ito (Goldman Sachs International, Global Markets), Giuliano De Rossi (Goldman Sachs International, Global Markets), Michael Steliaros (Goldman Sachs International, Global Markets)
ABSTRACT
We examine the relation between news arrival intensity, volatility and volume at an intraday frequency using a global dataset. The analysis is based on news analytics platforms that use natural language processing to perform entity recognition, classification by topic and sentiment analysis. We introduce our own news arrival intensity metric, which is simple and intuitive, and present compelling evidence that intraday volume and volatility forecasts can be improved using these metrics. For stocks traded in the U.S. and Europe, we use Refinitiv’s news analytics dataset based on news written in English. We present strong and robust out-of-sample performance of our model in these markets. The results for the U.S. and Europe suggest that it is possible to extract stronger signals from news articles written in languages that are native to each market. For this reason, we extend our model to stocks traded in Japan using a news analytics dataset provided by FTRI/Alexandria. This dataset is based on articles in Japanese published by Nikkei. Our model successfully harnesses markedly strong predictive power of news in this application. In particular, our out-of-sample analysis for Japanese stocks shows that about 80%∼90% of the stocks would have benefitted from the use of our news arrival intensity metrics. Our results also suggest a spillover effect within sectors: Volatility in stock i tends to increase if other companies in the same sector experience an increase in news arrival intensity. We demonstrate that the output of the model is economically and statistically significant and remains robust over time even in the presence of outlying data points. Our model can be applied to optimal trade execution both at the stock and at the portfolio level.
Chapter 15. Exogenous Risks Alternative Data Implications for Strategic Asset Allocation – Multi-Subordination Levy Processes Approach
Boryana Racheva-Iotova (FactSet)
ABSTRACT
The 21st century has already been marked by three fundamental paradigm shifts related to how we understand and model financial markets behavior – the incorporation of non-gaussian processes to represent extreme market events, MPT modification to account for behavioral biases and market participants’ preferences, and most recently – the need to include exogenous factors into the modeling considerations. The latter can be representative of the so-called novel risk factors which arise from environmental-, governance-, healthcare-, political-, policy-, technology-related and other similar potential disruptions, and can be characterized by (1) being factors external to the financial system itself, (2) requirements to be assessed based on alternative data, (3) not yet been priced by the market or, in other words, the markets are effectively not-yet-efficient with regards to these novel phenomena. While the first of the above-mentioned fundamental shifts has well-developed theoretical modeling foundations and the second one accumulates a body of research, the study of the latter one is still a fundamentally open question. Within this chapter, we will offer a general framework for modeling exogenous novel risk factors in an integrated framework via the notion of multi-subordinated Lťevy processes. The approach introduces a unified framework for consistent integration of traditional and novel types of risk and can serve for both risk budgeting and asset-allocation applications.
(PART IV.C) CASE STUDIES ON ESG
Chapter 16. ESG Controversies and Stock Returns
Tiago Quevedo Teodoro (MarketPsych Data), Joshua Clark-Bell (MarketPsych Data), Richard L. Peterson (MarketPsych Data)
ABSTRACT
In the chatter of online communities and in the writings of investigative journalists, corporate controversies are revealed and discussed in real time. Many of these controversies involve violations of environmental, social, and governance (ESG) standards. This paper studies the Refinitiv MarketPsych ESG Analytics, a dataset of ESG controversies extracted from real-time news articles and social media posts. Using monthly rotation models on the Russell 3000 constituents our research demonstrates that companies associated with a higher level of controversial online chatter experience greater future volatility and stock price underperformance. After excluding companies that are the most involved in ESG controversies, and controlling for the industry, a long-only simulated portfolio achieved annualized risk-adjusted returns 46% higher than the benchmark in the period from 2006 to 2020.
Chapter 17. Oil and Gas Drilling Waste – A Material Externality
J. Blake Scott (President, Waste Analytics LLC)
ABSTRACT
Waste is one of the issues mentioned in the United Nation’s Sustainable Development Goals (SDGs). A waste stream can be a material issue depending upon the volume of the waste generated, if the contaminants in the waste are of concern, and/or if it is poorly managed. Unfortunately, even material waste streams are usually not discussed in detail in corporate sustainability reporting or corporate financial reporting. These material, non-reported waste streams are externalities that society is unaware of their impacts. An example of a material waste that has a tremendous impact and that is not properly disclosed is oil and gas drilling waste. This case study will explore how drilling waste is a material externality, how it is not properly reported in corporate sustainability reporting, and the potential impacts to society if it is not properly addressed.
Chapter 18. ESG Scores and Price Momentum Are Compatible: Revisited
Matus Padysak (Quantpedia.com)
ABSTRACT
In recent years the ESG has become a very important topic area for the investment community and other financial market participants. Investment in assets which encourage sustainable developments is gaining more and more acceptance. We revisit well established research results of ESG and momentum investing. We then explore the scope of combining ESG style investing with a premier momentum-based trend. The theoretical background lies in the unbounded Knapsack problem; these genres of problems are solved using linear programming. The ESG implementation does not necessarily hamper the performance, and the long-only ESG portfolios turn out to be less risky. The risk-reducing effect, however, does not materialize significantly in the momentum-tilted portfolios.
PART (V) DIRECTORY OF ALTERNATIVE DATA VENDORS
ALEXANDRIA TECHNOLOGY
BLOOMBERG L.P
CHINASCOPE
EXANTE DATA
FACTEUS
FACTSET
GTCOM Technology Corporation
INFOTRIE FINANCIAL SOLUTIONS
MARKETPSYCH DATA
NIKKEI FTRI
NOWCAST INC.
RAVENPACK
REFINITIV
Ernest Chan
Respected Academic, Author, Practicing Fund Manager
Entrepreneur and Founder of PredictNow.AI
An impressive and timely contribution to the fast-developing discipline of data driven decisions in the trading and management of financial risk. Automated data collection, organization, and dissemination are part and parcel of Data Science and the Handbook covers the current breadth of these activities, their risks, rewards, and costs. A welcome addition to the landscape of quantitative finance.
Professor Dilip Madan
Professor of Finance, Robert H. Smith School of Business
Professor Gautam Mitra and his team unpack the topic of alternative data in finance, an ambitious endeavour given the fast-expanding nature of this new and exciting space. Alternative data powered by Natural Language Processing and Machine Learning has emerged as a new source of insights that can help investors make more informed decisions, stay ahead of competition and mitigate emerging risks. This handbook provides a strong validation of the substantial added value that alternative data brings. It also helps promote the idea that data driven decisions are better and more sustainable – something we, at RavenPack, firmly believe.
Armando Gonzalez
CEO and Founder of RavenPack
As the 1st Duke of Marlborough, John Churchill, wrote in 1715: ‘No war can be conducted successfully without early and good intelligence.’ The same can be said for successful trading. In that light, the Handbook of Alternative Data in Finance contains vital insights about how to gather and use alternative data — in short, intelligence — to facilitate successful trading.
Professor Steve H. Hanke
Professor of Applied Economics, The Johns Hopkins University
Baltimore, USA
Alternative data has become a hot topic in finance. New kinds of data, new data sources, and of course new tools for processing such data offer the possibility of new and previously unsuspected signals. In short alternative data lead to the promise of enhanced predictive power. But such advance does not come without its challenges – in terms of the quality of the data, the length of its history, reliable data capture, the development of appropriate statistical, AI, machine learning, and data mining tools, and, of course, the ethical challenges in the face of increasingly tough data protection regimes. Gautam Mitra and his colleagues have put together a superb collection of chapters discussing these topics, and more, to show how alternative data, used with care and expertise, can reveal the bigger picture.
Professor David J. Hand
Emeritus Professor of Mathematics and Senior Research Investigator,
Imperial College, London
Digital capital is now so important that it can rightly be viewed as a factor of production, especially in the financial sector. This handbook does for the field of alternative data what vendors of alternative data do for data itself; and that is to provide structure, filter noise, and bring clarity. It is an indispensable work which every financial professional can consult, be it for an overview of the field or for specific details about alternative data.
Professor Hersh Shefrin
Mario L. Belotti Professor of Finance, Santa Clara University
Use the Online Order Form
PHONE your credit card order:
+44 (0) 1895 256 484
You can also use the order form in the flyer and submit it one of the following ways:
EMAIL:
info@optirisk-systems.com
Managed by
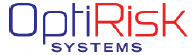